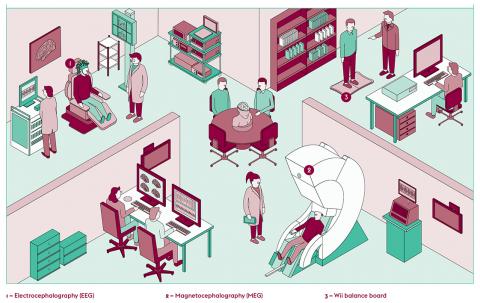
Algorithms and the human brain (L'Edition #12)
(Article from L'Edition N°12 - march 2020)
Statistical methods inspired by machine learning are becoming increasingly relevant to predicting or quantifying neurological disorders. The interdisciplinary research projects are exploring the human brain within the body envelope in a clinical context.
The scope of applications for machine learning, a specific branch of artificial intelligence, continues to expand as algorithms make their way into hospitals and specialised health departments. These information systems can analyse large quantities of data, detect common patterns and learn from examples as well as predict or quantify behaviours, results and tendencies, thus providing clinicians with valuable assistance in establishing their diagnoses.
These modern tools are vital to the work of Nicolas Vayatis, director of the new Centre Borelli, where they are implemented in a clinical context. On January 1, 2020, two research entities merged to form this interdisciplinary laboratory, namely, the Centre for Mathematical Studies and their Applications (CMLA – Université Paris-Saclay, ENS Paris-Saclay, CNRS) and the Cognition Action Group (“Cognac G” – Université de Paris, CNRS, French Military Health Service). These two teams, which include mathematicians, engineers, computer scientists, neurophysiologists and clinicians, have been working closely for several years, so the merger simply makes their cooperation official while promising higher visibility.
The research of these teams bears on the quantification of sensorimotricity (e.g. spatial displacements, gestures and eye movements) in human subjects in a healthy, altered or pathological state – e.g. affected by neurological disorders – for the purpose of developing methods that will optimise clinical validation. “The conventional validation process relies on simple statistical tests that were invented nearly one hundred years ago and are generally applied to small-scale samples. A very small number of variables are analysed separately to detect differences between patient populations,” recalls Nicolas Vayatis. “However, this method fails to detect differences that solely appear when conjoint analysis is used to study the variables.” Naturally, the mathematician and his colleagues are eager to exploit all of the dependencies using machine learning and its algorithms. “However, the strength of our work is based on accessing field data and on the support of neurophysiologists and clinicians who guide the quantitative part of the work,” adds Nicolas Vayatis.
Improving the ergonomics of quantitative measurement
Recently, the researchers collaborated with a French military teaching hospital, Hôpital d’Instruction des Armées de Percy, to conduct static balance analysis on patients presenting parkinsonian syndromes. The postural instability of such patients is often a major cause of disability due to falls. Generally, clinicians have relied on observation and everyday experience to determine a patient’s propensity to fall and establish a diagnosis during the medical consultation. Today, force platforms – whose price tag is generally high – are sometimes used to register the displacement of the centre of pressure of a patient standing erect and to produce statokinesigrams.
“We decided to use a Wii balance board – a much less expensive force platform – for the quantification of static balance,” reports Nicolas Vayatis. His team’s research was based on data from 123 patients with parkinsonian syndromes. The latter had been divided into two groups, those that did or did not have a propensity to fall, determined by means of a clinical assessment and the performance of a simple Romberg test. The team then devised a statistical method able to analyse statokinesigrams, yield a large number of posturographic characteristics after appropriate signal processing and detect significant differences between the two groups of patients
“By accessing and preprocessing the raw signal, which is very rich, and by controlling the entire signal processing chain, we were able to boost the number of measured parameters available for static analysis to nearly 1,000. When studying a particular pathology, some but not all of these parameters will be relevant. Working hand in hand with clinicians helps us interpret them and cluster them into a readily understandable language.”
For this kind of approach to succeed, the neurologists and clinicians must be familiar with the entire measurement and data processing chain. “The point is not to provide an automated diagnosis, but to provide a method compatible with their practice that will help them objectify their observations,” comments Nicolas Vayatis. Ergonomics was another key concern. For instance, the portable tablet, its application and its interface were designed for easy use during routine medical consultations. At the Centre Borelli, a dozen clinicians are already working with the prototypes developed thus far. Thanks to support from the French tech transfer company Erganeo, formerly known as SATT IDF INNOV, several patents bearing on these technologies have been obtained and led to licensing agreements with industrial firms. The next step is to formulate a business model and see that these technologies are added to the list of medical acts reimbursed by France’s national health authority.
Non-invasive electrophysiology and brain health
On another research front, the psychologist Denis Engemann is using non-invasive electrophysiology to explore disorders of consciousness. “Every time we tackle a particular disorder, we get a new perspective on the brain and how it works,” he says. A member of a joint Inria-CEA team at the NeuroSpin research centre (Université Paris-Saclay and CEA), he uses electrocephalography (EEG) and magnetocephalography (MEG) to study brain activity and machine learning to predict biomedical results. These two, complementary electrophysiological tests monitor the electrical activity of large neuronal assemblies in real time and yield, thanks to the studied biomarkers, unique insights into brain health and states of consciousness. “For instance, a change in alpha power is the signature of a change in consciousness, which may be found in every EEG recording done for a human being,” he explains.
Given the complexity of MEG/EEG data (e.g. power of the signal and frequency peaks), Denis Engemann develops specific applications and algorithms able to yield representations that will be useful in creating statistical models. “One of our aims is to successfully predict the relationship between the MEG/EEG signals and one of the patient’s health parameters.” While the MEG and EEG are governed by the same biophysical principles, they present differences when it comes to data analysis. “Since EEG signals are weaker than MEG signals, they are more vulnerable to electrical noise, i.e. electromagnetic interference, in the environment. It will be important to develop specific signal processing methods that will clean up signals automatically and isolate the signal of interest.”
Shedding light on anaesthesia
As part of a recent collaboration with Hôpital de Lariboisière in Paris, Denis Engemann and his team focused on the connection between anaesthesia and aging. “Understanding how to automate the dose of anaesthetic administered to a patient in the operating room is of both practical and social import,” he remarks. Before a surgical procedure, the anaesthesiologist administers a drug so that the patient will lose consciousness and remain immobile and the surgical team can operate without inflicting pain. Throughout the surgical act, the patient’s physiological parameters (e.g. cardiac frequency, blood pressure and oxygen saturation) are observed. When these parameters are no longer optimal, the practitioner often intuits, based on past experience, how to adapt the dose of anaesthetic as a function of the patient’s gender or age. “Our team is developing statistical methods that try to succeed in automating these intuitions.”
Anaesthesia has been identified as a probable cause of some postoperative problems. “Our job is to find out the connections between the drugs, their physiological effects, the depth of the anaesthesia and the outcome of the operation. Knowing how physiological age, brain age and brain signal relate to each other makes it possible to predict the amount and type of medication required.”
Recently, Denis Engemann developed statistical models that used MEG recordings combined with neuroimaging (MRI and fMRI) to predict a person’s age. He relied on raw data on 650 people published by the Cambridge Centre for Ageing and Neuroscience. “The idea is to see how the prediction of age pertains to the appearance of neurodegenerative disorders.” In the course of the study, he noted residual variance between predicted age and physiological age. “Persons whose predicted age was exaggerated had weaker cognitive abilities than the others,” says Denis Engemann. At any event, one thing is certain: the process of crossinspiration between the neurosciences and machine learning is just getting started!
Publications
∙ Denis Engemann et al., Combining electrophysiology with MRI enhances learning of surrogate-biomarkers. November 2019.
∙ Nicolas Vayatis et al., Revealing posturographic features associated with the risk of falling in patients with Parkinsonian syndromes via machine learning.