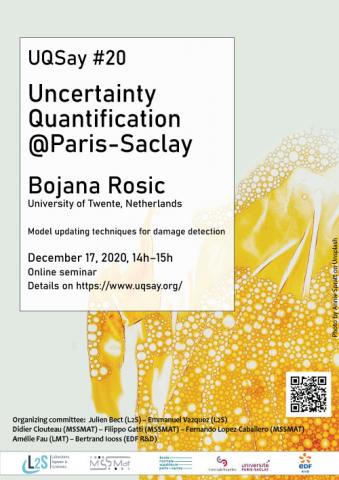
UQSay seminar #20: Model updating techniques for damage detection
One of the main issues in material science is estimation of the constitutive laws given experimental data that may come in different forms ranging from the microscopic images to the macroscopic data collected by strain gauges for example. As data are often heterogeneous, of multi-scale/temporal nature, possibly ambiguous and of low quality due to missing values, the process of learning is often requiring the careful application of existing or design of new data fusion algorithms that are bounded to small data sets. In this talk will be presented the computationally efficient Bayesian algorithms for the damage estimation. In particular, the special attention will be paid to damage model estimation by using both classical uncertainty quantification as well as machine/deep learning approaches.
Joint work with (alphabetical order) X. Chapeleau, P.-E. Charbonnel, L.-M. Cottineau, L. De Lorenzis, A. Ibrahimbegovic, V. Le Corvec, H.G. Matthies, E. Merliot, M.S. Sarfaraz, D. Siegert, R. Vidal, J. Waeytens and T. Wu.
Gif-sur-YvetteOne of the main issues in material science is estimation of the constitutive laws given experimental data that may come in different forms ranging from the microscopic images to the macroscopic data collected by strain gauges for example. As data are often heterogeneous, of multi-scale/temporal nature, possibly ambiguous and of low quality due to missing values, the process of learning is often requiring the careful application of existing or design of new data fusion algorithms that are bounded to small data sets. In this talk will be presented the computationally efficient Bayesian algorithms for the damage estimation. In particular, the special attention will be paid to damage model estimation by using both classical uncertainty quantification as well as machine/deep learning approaches.
Joint work with (alphabetical order) X. Chapeleau, P.-E. Charbonnel, L.-M. Cottineau, L. De Lorenzis, A. Ibrahimbegovic, V. Le Corvec, H.G. Matthies, E. Merliot, M.S. Sarfaraz, D. Siegert, R. Vidal, J. Waeytens and T. Wu.