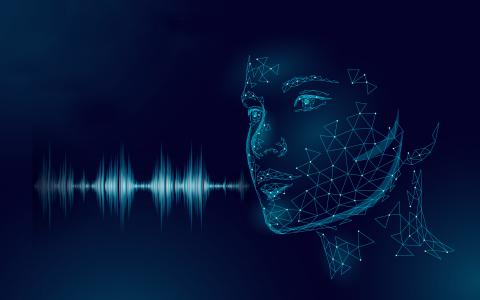
Will language be deciphered by new technologies?
How is language processed? This question, first tackled by linguists and philosophers, and then by neuroscientists, has fascinated scientists for a long time. Today, research on this subject is progressing in leaps and bounds thanks to the development of new technologies such as brain imaging, genomics, artificial intelligence and machine translation, etc. Researchers in the laboratories at Université Paris-Saclay are dissecting every aspect of language in order to understand its very essence and to develop tools for society.
Most living things can communicate with each other using warning messages or emotional signals. Only humans, however, have the cognitive functions to express complex and abstract ideas through language. Biologists are trying to explain this unique characteristic using the human genome. They think that this ability to communicate originates in the DNA of the cell nucleus. This acts as a carrier for the genetic information which shapes the body as well as its organs and their capacities.
What are the language genes?
Although scientists have identified the location of most human genes on chromosomes, they do not yet know how they all work, and this applies to those linked with language in particular. In order to fill this gap, the Brainomics team at the NeuroSpin (Univ. Paris-Saclay, CEA) laboratory are using the very new technology of genetic imaging. This tracks morphological and functional features in the brain and links them to genome variations in the human population.
It has been known for a long time that language skills involve very specific areas of the brain. For example, depending on the area affected, some brain injuries lead to difficulties in reading, understanding and speaking. These areas can now be mapped at a very fine spatial and temporal resolution using brain imaging. Functional magnetic resonance imaging (fMRI) (one of the best-known imaging techniques) detects changes in oxygen levels in the brain using electromagnetic waves. Neurons located in active areas consume more oxygen and this highlights the brain activity.
The brain never stops talking
In their recent work, Cathy Philippe and Yasmina Mekki from Brainomics have observed areas of the brain using fMRI which are known to be linked with language. However, they have done this in a very particular context, i.e. when people are resting. “Even when the brain is not asked to carry out a cognitive function, activity can be observed in the neural network. This activity is weaker and more fleeting in resting state fMRI than in conventional fMRI, but the brain still shows some activity,” explains Cathy Philippe. The researchers have analysed the neural activity of 30,000 resting British individuals, together with genomic data, and made them available to scientists around the world.
Their statistical study investigated functional connectivity, with regions (such as areas involved in syntax and phonology) working together. The researchers have scrutinized over 300 examples of connectivities known to be linked to language. By cross-checking these elements with genomic mutations present in the participants (those linked to single nucleotide polymorphisms (SNPs)), they have identified the chromosomal positions of some twenty genes involved in language. “As language is a very complex process, many genes are associated with it,” points out Yasmina Mekki. Their work goes into even more detail. “We’ve linked the regulation of the EPHA3 gene to the semantic component of language,” say the researchers.
How do you know it is the end of a sentence?
These functional connectivities are of great importance as they highlight the brain’s incredible abilities. The organ carries out a multitude of tasks to do with syntax, phonology and semantics in the blink of an eye without humans feeling like they have made any effort at all. It is therefore quite logical that, since the 1940s, artificial intelligence (AI) has sought to reproduce the structure of the brain using the construction of artificial neural networks.
Society sees this as an indicator of the progress made by artificial intelligence as language is considered a marker of intelligence. The fantasy of a talking machine has endured, from the early days of computing and the invention of Alan Turing's imitation test, right up to the recent popularity of smartphones and connected speakers. This fascination has led to numerous advances in AI in the field of language, including automatic language processing, speech recognition, text synthesis and translation.
In order to carry out these developments, it is common to take a pre-existing model capable of analysing natural language texts as a starting point. A model such as this (BERT or GPT-2, for example), which is designed to mimic human linguistic performance to a greater or lesser extent, then goes through an unsupervised learning phase. Using a large body of texts, such as those on Wikipedia, it guesses the end of a sentence by accessing only the first few words. The machine then slowly makes connections between words and improves its ability to predict. The advantage of this approach is that it is immediately possible to compare the performance of the machine with that of a human. If only a few words are given, for example ‘the dog...’ the task is more complicated for both the machine and the human. However, the more information both receive, the higher the success rate becomes. If a sentence starts with ‘The dog chased the...’, a powerful and sufficiently trained model is able to determine that the combination of words ‘dog’ and ‘chased’ are often found with the word ‘cat’.
A useful dialogue between humans and machines
This does not mean that everything has been solved. “Even if humans and machines come to similarconclusion, can it be said that they have used the same intermediate processes to get there?” asks Charlotte Caucheteux and Alexandre Gramfort. Working together with the MIND team (Univ. Paris-Saclay, Inria, CEA) which is a part of NeuroSpin, the two researchers are comparing human brains and artificial neural networks. Using fMRI, they have created a spatial map of the brain and supplemented it with a temporal analysis provided by magnetoencephalography (MEG) which captures the magnetic fields generated by the neurons. “We still don’t fully understand the intermediate representations made by the brain during language processing,” explain the two scientists. “But we have divided the cognitive process into three stages of increasing abstraction.”
When a sentence is read, the visual level is activated first and this takes place in the first hundred milliseconds. Then the lexical level identifies the word which has just been read. Finally, the compositional level places the word in the context of the sentence. “We show that these three levels of representation correspond to three types of algorithm: convolutional image processing algorithms, lexical embedding algorithm, and langage models like GPT-2,” Charlotte Caucheteux reveals.
Scientists in the MIND team are modifying neural network architectures and long-term prediction training tasks in order to be able to design ever more brain-like algorithms. As Alexandre Gramfort explains, “The interaction between neuroscience and AI is a two-way process. AI can model the human brain, reducing reliance on time-consuming and expensive brain imaging studies. In turn, a better understanding of the brain helps us to improve AI.”
Finding the right match
The interactions and similarities between machines and humans do not end there. “The human brain, just like these algorithms, is always trying to predict the future - the end of a sentence, the rest of a story, etc.” points out Yair Lakretz, who works at the UNICOG team at NeuroSpin and who studies the similarities between humans and machines. He believes that observing the most efficient algorithms could reveal what is going on in human heads. “Biologists study laboratory mice to understand blood circulation or diabetes. But animal models don’t exist for language. So, why not create an artificial neural model instead?” suggests the researcher.
After having trained his models to complete sentences, Yair Lakretz confronts them with another challenge, namely that of grammatical agreement quizzes. The models he studies are generally quite good for sentences containing a single dependency. They choose, for example ‘the keys that the man is holding are...’ instead of ‘The keys that the man is holding is...’ and are successful at identifying the subject with which the verb agrees, even if several words separate them.
According to the researchers at NeuroSpin, artificial neural models remember words at the beginning of a sentence in a similar way to the human brain when an individual is reading a text. However, if they are given a more complex sentence, such as ‘The keys which the man who is near the table is holding...’ the models just give up. This is because they have only two memory units which are quickly overloaded, making them very unstable. In contrast, with humans any loss in efficiency is more gradual. They make mistakes, but always answer correctly in over half of cases.
“Even though models are a good approximation, there’s potentially something very different in the way algorithms and humans process language,” qualifies the researcher. While it’s amazing to see the similarities between artificial and human neural networks, it’s equally exciting to look at their differences.”
The recursivity of human thought
When it comes to the sentence ‘She belonged to that half of the human race in whom the curiosity the other half feels about the people it does not know is replaced by an interest in the people it does’, a human being is able to understand this complex syntax by re-reading it, if necessary, several times. Models are not ready to do this - a bit more time will be needed before they can tackle texts by Marcel Proust!
“The human brain's understanding of language relies on its ability to be recursive,” says Yair Lakretz of NeuroSpin. Recursivity is the ability to repeat a structure within the same structure. For example, the statement ‘The car is red’ may contain a second one, i.e. ‘The car parked in front of the house is red’. “Recursivity is a constituent part of human languages, even if it’s more apparent in some than in others, such as French sign language,” explains Michael Filhol from the Information, Written and Signed Language team (ILES) at Interdisciplinary Laboratory of Digital Sciences (LISN - Univ. Paris-Saclay, CNRS, CentraleSupélec, Inria).
This is where the difference between humans and machines lies. “Humans have the ability to understand a highly recursive sentence, but are limited by the capacity of their short-term working memory,” suggests Yair Lakretz de NeuroSpin. In contrast, the underperformance of the models can be explained by their inability to handle recursion. If AI could one day acquire this skill, it will certainly become more efficient and even perhaps understand literary texts fully.
Language, languages and translation
Another striking fact is that the universal cognitive capacity of language in human beings is expressed in a multitude of languages which have emerged to suit local needs and cultures. As scientists cannot study them all, they have chosen one or a small number of languages around which to focus their work. Charlotte Caucheteux and Alexandre Gramfort train their algorithms in Dutch and Yair Lakretz trains his in English and Italian. “Even if the majority of studies focus on English (the international language of research), it’s interesting to study linguistic peculiarities, such as gender, in French, or compound words, in German,” points out Yair Lakretz.
A whole area of research is concerned with translation, and thanks to technological advances, this work no longer rests solely on the shoulders of interpreters and translators. Automatic language processing has enabled the development of online translation tools used by millions of people. The scientists at Université Paris Saclay are making their own contribution, alongside large Silicon Valley companies such as GAFAM. “The ultimate goal is to create algorithms which can be given any written text or audio extract and be able to translate it into a spoken or written form. At LISN, we assess the quality of existing algorithms which perform, for example, tasks of translation and automatic text alignment. We’re also designing new neural network architectures for multilingual tasks,” explains François Yvon, who is part of the Processing of Spoken Language team(TLP) at LISN and a specialist in this subject area.
Languages on the verge of disappearing
François Yvon is particularly interested in the preservation of endangered languages. Computer scientists and linguists are working together to document these little-known languages for which often only fragments of translation exist. Linguists know how to identify their similarities and regular patterns in order to decipher their overall structure, but this work is long and tedious. Algorithms can now assist specialists in their work by transcribing a recording into phonetic notation and then delineating semantic units. Each algorithm transcribes an audio document into meaningful written words which are easier to analyse. The AI does not work alone but makes the work of linguists easier in that they only have to check the validity of the automatic annotations delivered by the machine.
An avatar which speaks using signs
In the case of French Sign Language (LSF), which is much more widely used than these endangered languages, no written notation system has been adopted by the community. “Three options currently exist for writing down anything communicated using LSF. It can be written in written French, but this requires quite considerable translation skills, and the characteristics of LSF, such as its syntax or recursivity, are lost. It’s also possible to use one of the existing official sign language writing systems, which aren’t widely used in practice. The third option is to use a personal notation,” mentions Michael Filhol. The researcher has compiled several examples of spontaneous notes made by individuals. While existing systems are only phonographic and transcribe arm, hand or face movements into pictograms, personal notes integrate a significant number of logographic symbols, i.e. related to the meaning of words.
On the basis of this observation, the researcher and his team are creating a new graphic formalisation in collaboration with speakers of LSF. Ultimately, it will be used in software similar to word processing softwares or to produce a reading by an animated avatar. Michael Filhol is already working with a research team in Chicago on the development of an avatar called Paula to produce sign language as naturally as possible.
The research being carried out aims to meet many of society's demands through combining language and technology. It will continue to offer answers to human cognitive mechanisms, and new tools to better understand language.
Publications:
- Yasmina Mekki et al. "The genetic architecture of language functional connectivity." NeuroImage vol. 249 (2022): 118795.
- Caucheteux, C., King, JR. Brains and algorithms partially converge in natural language processing. Communications Biology 5, 134 (2022).
- Charlotte Caucheteux, Alexandre Gramfort, and Jean-Remi King. Disentangling syntax and semantics in the brain with deep networks. International Conference on Machine Learning. PMLR, (2021).
- Yair Lakretz, Dieuwke Hupkes, Alessandra Vergallito, Marco Marelli, Marco Baroni, Stanislas Dehaene. Mechanisms for handling nested dependencies in neural-network language models and humans. Cognition, Volume 213, 2021, 104699, ISSN 0010-0277.
- Gilles Adda, Sebastian Stüker, Martine Adda-Decker, Odette Ambouroue, Laurent Besacier, David Blachon, Hélène Bonneau-Maynard, Pierre Godard, Fatima Hamlaoui, Dmitry Idiatov, Guy-Noël Kouarata, Lori Lamel, Emmanuel-Moselly Makasso, Annie Rialland, Mark Van de Velde, François Yvon, Sabine Zerbian. Breaking the Unwritten Language Barrier: The BULB Project. Procedia Computer Science, Volume 81, 2016, Pages 8-14, ISSN 1877-0509.
- McDonald, J., Filhol, M. Natural synthesis of productive forms from structured descriptions of sign language. Machine Translation 35, 363–386 (2021).