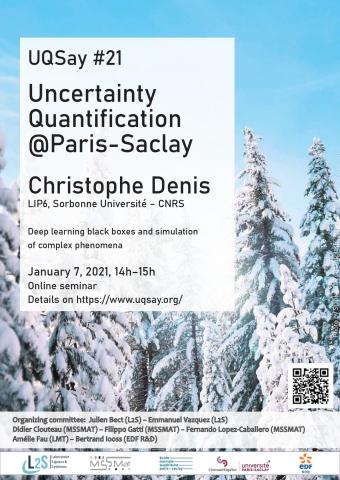
UQSay Seminar #21: Deep learning black boxes and simulation of complex phenomena
The joint scientific advances on mathematical modeling and on high performance computing has increased for decades the precision of digital simulations. These simulations are built on a hypothetico-deductive model in which the phenomena behavior is governed by mathematical equations. Since 2010, Artificial Intelligence based on Machine Learning has produced impressive results, mainly in the fields of the pattern recognition and the natural language processing, succeeding to the previous dominance of the symbolic AI, centered on the logical reasoning. The integration of ML methods into industrial processes gives hope for new growth drivers. At the scientific level, the use of Deep Learning is an alternative to predict complex phenomena suffering from epistemic uncertainties in the hope of improving the scientific knowledge. A major obstacle remains the lack of validation and explainability of machine learning application. It is therefore necessary to produce ethical explanations of predictions adapted to recipients. It is also necessary to define an epistemological model of deep learning since it calls into question certain paradigms of classical statistical theory.
This talk presents the main lines of our research project done in collaboration with philosophers of science. The main objective is to be able to use Deep Learning to generate new scientific knowledge for example on chaotic systems in climate modelling. The first step of this project, done with Franck Varenne, has been to underline the epistemic differences between of a machine learning model and a causal mathematical model. The second part of the talk deals with the multidimensional evaluation of explanations generated from outcomes of a machine learning application.
4, avenue des sciences Gif-sur-YvetteThe joint scientific advances on mathematical modeling and on high performance computing has increased for decades the precision of digital simulations. These simulations are built on a hypothetico-deductive model in which the phenomena behavior is governed by mathematical equations. Since 2010, Artificial Intelligence based on Machine Learning has produced impressive results, mainly in the fields of the pattern recognition and the natural language processing, succeeding to the previous dominance of the symbolic AI, centered on the logical reasoning. The integration of ML methods into industrial processes gives hope for new growth drivers. At the scientific level, the use of Deep Learning is an alternative to predict complex phenomena suffering from epistemic uncertainties in the hope of improving the scientific knowledge. A major obstacle remains the lack of validation and explainability of machine learning application. It is therefore necessary to produce ethical explanations of predictions adapted to recipients. It is also necessary to define an epistemological model of deep learning since it calls into question certain paradigms of classical statistical theory.
This talk presents the main lines of our research project done in collaboration with philosophers of science. The main objective is to be able to use Deep Learning to generate new scientific knowledge for example on chaotic systems in climate modelling. The first step of this project, done with Franck Varenne, has been to underline the epistemic differences between of a machine learning model and a causal mathematical model. The second part of the talk deals with the multidimensional evaluation of explanations generated from outcomes of a machine learning application.