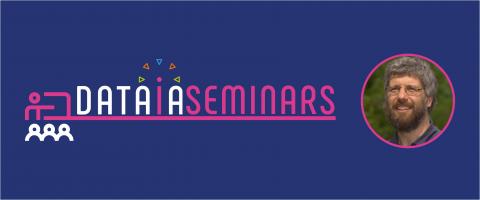
24.09.20 DATAIA Seminar | « Solving inverse problems with invertible neural networks » - Ulrich Köthe
Ulrich Köthe (Université de Heidelberg) est l’animateur du séminaire DATAIA du mois de septembre, sur le theme « Solving inverse problems with invertible neural networks ».
Resumé :
Interpretable models are a hot topic in neural network research. This talk will focus on inverse problems, where one wants to infer backwards from observations to the hidden characteristics of a system. I will focus on three aspects of interpretability: reliable uncertainty quantification, outlier detection, and disentanglement into meaningful features. It turns out that invertible neural networks -- networks that work equally well in the forward and inverse direction -- are great tools for that kind of analysis: They act as non-linear generalizations of classical methods like PCA and ICA. Examples from physics, medicine, and computer vision demonstrate the practical utility of the new method.
Le séminaire aura lieu en ligne. Cliquez ici pour accéder au webinaire.
chez soiUlrich Köthe (Université de Heidelberg) est l’animateur du séminaire DATAIA du mois de septembre, sur le theme « Solving inverse problems with invertible neural networks ».
Resumé :
Interpretable models are a hot topic in neural network research. This talk will focus on inverse problems, where one wants to infer backwards from observations to the hidden characteristics of a system. I will focus on three aspects of interpretability: reliable uncertainty quantification, outlier detection, and disentanglement into meaningful features. It turns out that invertible neural networks -- networks that work equally well in the forward and inverse direction -- are great tools for that kind of analysis: They act as non-linear generalizations of classical methods like PCA and ICA. Examples from physics, medicine, and computer vision demonstrate the practical utility of the new method.
Le séminaire aura lieu en ligne. Cliquez ici pour accéder au webinaire.